mirror of
https://github.com/ClickHouse/ClickHouse.git
synced 2024-11-18 05:32:52 +00:00
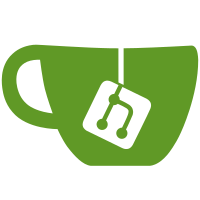
* CLICKHOUSE-4063: less manual html @ index.md * CLICKHOUSE-4063: recommend markdown="1" in README.md * CLICKHOUSE-4003: manually purge custom.css for now * CLICKHOUSE-4064: expand <details> before any print (including to pdf) * CLICKHOUSE-3927: rearrange interfaces/formats.md a bit * CLICKHOUSE-3306: add few http headers * Remove copy-paste introduced in #3392 * Hopefully better chinese fonts #3392 * get rid of tabs @ custom.css * Apply comments and patch from #3384 * Add jdbc.md to ToC and some translation, though it still looks badly incomplete * minor punctuation * Add some backlinks to official website from mirrors that just blindly take markdown sources * Do not make fonts extra light * find . -name '*.md' -type f | xargs -I{} perl -pi -e 's//g' {} * find . -name '*.md' -type f | xargs -I{} perl -pi -e 's/ sql/g' {} * Remove outdated stuff from roadmap.md * Not so light font on front page too * Refactor Chinese formats.md to match recent changes in other languages
16 lines
1.7 KiB
Markdown
16 lines
1.7 KiB
Markdown
# General Questions
|
|
|
|
## Why Not Use Something Like MapReduce?
|
|
|
|
We can refer to systems like MapReduce as distributed computing systems in which the reduce operation is based on distributed sorting. The most common open source solution in this class is [Apache Hadoop](http://hadoop.apache.org). Yandex uses their in-house solution, YT.
|
|
|
|
These systems aren't appropriate for online queries due to their high latency. In other words, they can't be used as the back-end for a web interface.
|
|
These types of systems aren't useful for real-time data updates.
|
|
Distributed sorting isn't the best way to perform reduce operations if the result of the operation and all the intermediate results (if there are any) are located in the RAM of a single server, which is usually the case for online queries. In such a case, a hash table is the optimal way to perform reduce operations. A common approach to optimizing map-reduce tasks is pre-aggregation (partial reduce) using a hash table in RAM. The user performs this optimization manually.
|
|
Distributed sorting is one of the main causes of reduced performance when running simple map-reduce tasks.
|
|
|
|
Most MapReduce implementations allow you to execute arbitrary code on a cluster. But a declarative query language is better suited to OLAP in order to run experiments quickly. For example, Hadoop has Hive and Pig. Also consider Cloudera Impala or Shark (outdated) for Spark, as well as Spark SQL, Presto, and Apache Drill. Performance when running such tasks is highly sub-optimal compared to specialized systems, but relatively high latency makes it unrealistic to use these systems as the backend for a web interface.
|
|
|
|
|
|
[Original article](https://clickhouse.yandex/docs/en/faq/general/) <!--hide-->
|