mirror of
https://github.com/ClickHouse/ClickHouse.git
synced 2024-11-18 05:32:52 +00:00
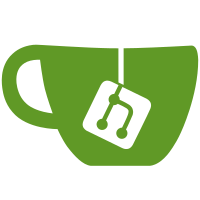
* Doc fix: add spaces where missing
* Doc fixes: rm double spaces
* Doc fixes: edit spaces
* Doc fixes: rm double spaces in /fa
* Revert "Doc fixes: rm double spaces in /fa"
This reverts commit bb879a62ef
.
* Doc fix: resolve all problems with double spaces in /fa
* Doc fix: add spaces for readability
* Doc fix: add spaces
* Fix spaces
13 lines
1.4 KiB
Markdown
13 lines
1.4 KiB
Markdown
# 常见问题
|
||
|
||
## 为什么不使用MapReduce之类的产品呢?
|
||
|
||
我们可以将MapReduce这类的系统称为分布式计算系统,其reduce操作基于分布式排序。其中最常见的开源解决方案是 [Apache Hadoop](http://hadoop.apache.org)。 Yandex使用他们的内部解决方案YT。
|
||
|
||
这些系统不适合在线查询,因为它们的延迟高。换句话说,它们不能用作Web接口的后端服务。这些系统对于实时数据更新是没有用的。如果操作的结果和所有中间结果(如果有的话)位于单个服务器的内存中,则分布式排序不是执行reduce操作的最佳方式,但这通常是在线查询的情况。在这种情况下,哈希表是执行reduce操作的最佳方式。优化map-reduce任务的常用方法是使用内存中的哈希表进行预聚合(部分reduce),用户手动执行此优化操作。分布式排序是运行简单map-reduce任务时性能降低的主要原因之一。
|
||
|
||
大多数MapReduce系统允许您在集群上执行任意代码。但是,声明性查询语言更适合OLAP,以便快速运行实验。例如,Hadoop包含Hive和Pig,Cloudera Impala或Shark(过时)for Spark,以及Spark SQL、Presto和Apache Drill。与专业系统相比,运行此类任务时的性能非常不理想,所以将这些系统用作Web接口的后端服务是不现实的,因为延迟相对较高。
|
||
|
||
|
||
[来源文章](https://clickhouse.yandex/docs/zh/faq/general/) <!--hide-->
|