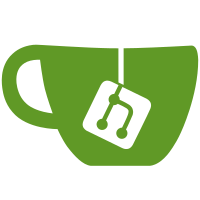
* CLICKHOUSE-4063: less manual html @ index.md * CLICKHOUSE-4063: recommend markdown="1" in README.md * CLICKHOUSE-4003: manually purge custom.css for now * CLICKHOUSE-4064: expand <details> before any print (including to pdf) * CLICKHOUSE-3927: rearrange interfaces/formats.md a bit * CLICKHOUSE-3306: add few http headers * Remove copy-paste introduced in #3392 * Hopefully better chinese fonts #3392 * get rid of tabs @ custom.css * Apply comments and patch from #3384 * Add jdbc.md to ToC and some translation, though it still looks badly incomplete * minor punctuation * Add some backlinks to official website from mirrors that just blindly take markdown sources * Do not make fonts extra light * find . -name '*.md' -type f | xargs -I{} perl -pi -e 's//g' {} * find . -name '*.md' -type f | xargs -I{} perl -pi -e 's/ sql/g' {} * Remove outdated stuff from roadmap.md * Not so light font on front page too * Refactor Chinese formats.md to match recent changes in other languages * Update some links on front page * Remove some outdated comment * Add twitter link to front page * More front page links tuning * Add Amsterdam meetup link * Smaller font to avoid second line * Add Amsterdam link to README.md * Proper docs nav translation * Back to 300 font-weight except Chinese * fix docs build * Update Amsterdam link * remove symlinks * more zh punctuation * apply lost comment by @zhang2014 * Apply comments by @zhang2014 from #3417 * Remove Beijing link * rm incorrect symlink * restore content of docs/zh/operations/table_engines/index.md * CLICKHOUSE-3751: stem terms while searching docs * CLICKHOUSE-3751: use English stemmer in non-English docs too * CLICKHOUSE-4135 fix * Remove past meetup link * Add blog link to top nav * Add ContentSquare article link * Add form link to front page + refactor some texts * couple markup fixes * minor * Introduce basic ODBC driver page in docs * More verbose 3rd party libs disclaimer * Put third-party stuff into a separate folder * Separate third-party stuff in ToC too * Update links * Move stuff that is not really (only) a client library into a separate page * Add clickhouse-hdfs-loader link * Some introduction for "interfaces" section * Rewrite tcp.md * http_interface.md -> http.md * fix link * Remove unconvenient error for now * try to guess anchor instead of failing * remove symlink * Remove outdated info from introduction * remove ru roadmap.md * replace ru roadmap.md with symlink * Update roadmap.md * lost file * Title case in toc_en.yml * Sync "Functions" ToC section with en * Remove reference to pretty old ClickHouse release from docs * couple lost symlinks in fa * Close quote in proper place * Rewrite en/getting_started/index.md * Sync en<>ru getting_started/index.md * minor changes * Some gui.md refactoring * Translate DataGrip section to ru * Translate DataGrip section to zh * Translate DataGrip section to fa * Translate DBeaver section to fa * Translate DBeaver section to zh * Split third-party GUI to open-source and commercial * Mention some RDBMS integrations + ad-hoc translation fixes * Add rel="external nofollow" to outgoing links from docs * Lost blank lines * Fix class name * More rel="external nofollow" * Apply suggestions by @sundy-li * Mobile version of front page improvements * test * test 2 * test 3 * Update LICENSE * minor docs fix * Highlight current article as suggested by @sundy-li * fix link destination * Introduce backup.md (only "en" for now) * Mention INSERT+SELECT in backup.md * Some improvements for replication.md * Add backup.md to toc * Mention clickhouse-backup tool * Mention LightHouse in third-party GUI list * Introduce interfaces/third-party/proxy.md * Add clickhouse-bulk to proxy.md * Major extension of integrations.md contents * fix link target * remove unneeded file * better toc item name * fix markdown * better ru punctuation * Add yet another possible backup approach * Simplify copying permalinks to headers * Support non-eng link anchors in docs + update some deps * Generate anchors for single-page mode automatically * Remove anchors to top of pages * Remove anchors that nobody links to * build fixes * fix few links * restore css * fix some links * restore gifs * fix lost words * more docs fixes * docs fixes * NULL anchor * update urllib3 dependency * more fixes
9.5 KiB
Storing Dictionaries in Memory
There are a variety of ways to store dictionaries in memory.
We recommend flat, hashed and complex_key_hashed. which provide optimal processing speed.
Caching is not recommended because of potentially poor performance and difficulties in selecting optimal parameters. Read more in the section "cache".
There are several ways to improve dictionary performance:
- Call the function for working with the dictionary after
GROUP BY
. - Mark attributes to extract as injective. An attribute is called injective if different attribute values correspond to different keys. So when
GROUP BY
uses a function that fetches an attribute value by the key, this function is automatically taken out ofGROUP BY
.
ClickHouse generates an exception for errors with dictionaries. Examples of errors:
- The dictionary being accessed could not be loaded.
- Error querying a
cached
dictionary.
You can view the list of external dictionaries and their statuses in the system.dictionaries
table.
The configuration looks like this:
<yandex>
<dictionary>
...
<layout>
<layout_type>
<!-- layout settings -->
</layout_type>
</layout>
...
</dictionary>
</yandex>
Ways to Store Dictionaries in Memory
flat
The dictionary is completely stored in memory in the form of flat arrays. How much memory does the dictionary use? The amount is proportional to the size of the largest key (in space used).
The dictionary key has the UInt64
type and the value is limited to 500,000. If a larger key is discovered when creating the dictionary, ClickHouse throws an exception and does not create the dictionary.
All types of sources are supported. When updating, data (from a file or from a table) is read in its entirety.
This method provides the best performance among all available methods of storing the dictionary.
Configuration example:
<layout>
<flat />
</layout>
hashed
The dictionary is completely stored in memory in the form of a hash table. The dictionary can contain any number of elements with any identifiers In practice, the number of keys can reach tens of millions of items.
All types of sources are supported. When updating, data (from a file or from a table) is read in its entirety.
Configuration example:
<layout>
<hashed />
</layout>
complex_key_hashed
This type of storage is for use with composite keys. Similar to hashed
.
Configuration example:
<layout>
<complex_key_hashed />
</layout>
range_hashed
The dictionary is stored in memory in the form of a hash table with an ordered array of ranges and their corresponding values.
This storage method works the same way as hashed and allows using date/time ranges in addition to the key, if they appear in the dictionary.
Example: The table contains discounts for each advertiser in the format:
+---------------+---------------------+-------------------+--------+
| advertiser id | discount start date | discount end date | amount |
+===============+=====================+===================+========+
| 123 | 2015-01-01 | 2015-01-15 | 0.15 |
+---------------+---------------------+-------------------+--------+
| 123 | 2015-01-16 | 2015-01-31 | 0.25 |
+---------------+---------------------+-------------------+--------+
| 456 | 2015-01-01 | 2015-01-15 | 0.05 |
+---------------+---------------------+-------------------+--------+
To use a sample for date ranges, define the range_min
and range_max
elements in the structure.
Example:
<structure>
<id>
<name>Id</name>
</id>
<range_min>
<name>first</name>
</range_min>
<range_max>
<name>last</name>
</range_max>
...
To work with these dictionaries, you need to pass an additional date argument to the dictGetT
function:
dictGetT('dict_name', 'attr_name', id, date)
This function returns the value for the specified id
s and the date range that includes the passed date.
Details of the algorithm:
- If the
id
is not found or a range is not found for theid
, it returns the default value for the dictionary. - If there are overlapping ranges, you can use any.
- If the range delimiter is
NULL
or an invalid date (such as 1900-01-01 or 2039-01-01), the range is left open. The range can be open on both sides.
Configuration example:
<yandex>
<dictionary>
...
<layout>
<range_hashed />
</layout>
<structure>
<id>
<name>Abcdef</name>
</id>
<range_min>
<name>StartDate</name>
</range_min>
<range_max>
<name>EndDate</name>
</range_max>
<attribute>
<name>XXXType</name>
<type>String</type>
<null_value />
</attribute>
</structure>
</dictionary>
</yandex>
cache
The dictionary is stored in a cache that has a fixed number of cells. These cells contain frequently used elements.
When searching for a dictionary, the cache is searched first. For each block of data, all keys that are not found in the cache or are outdated are requested from the source using SELECT attrs... FROM db.table WHERE id IN (k1, k2, ...)
. The received data is then written to the cache.
For cache dictionaries, the expiration lifetime of data in the cache can be set. If more time than lifetime
has passed since loading the data in a cell, the cell's value is not used, and it is re-requested the next time it needs to be used.
This is the least effective of all the ways to store dictionaries. The speed of the cache depends strongly on correct settings and the usage scenario. A cache type dictionary performs well only when the hit rates are high enough (recommended 99% and higher). You can view the average hit rate in the system.dictionaries
table.
To improve cache performance, use a subquery with LIMIT
, and call the function with the dictionary externally.
Supported sources: MySQL, ClickHouse, executable, HTTP.
Example of settings:
<layout>
<cache>
<!-- The size of the cache, in number of cells. Rounded up to a power of two. -->
<size_in_cells>1000000000</size_in_cells>
</cache>
</layout>
Set a large enough cache size. You need to experiment to select the number of cells:
- Set some value.
- Run queries until the cache is completely full.
- Assess memory consumption using the
system.dictionaries
table. - Increase or decrease the number of cells until the required memory consumption is reached.
!!! warning Do not use ClickHouse as a source, because it is slow to process queries with random reads.
complex_key_cache
This type of storage is for use with composite keys. Similar to cache
.
ip_trie
This type of storage is for mapping network prefixes (IP addresses) to metadata such as ASN.
Example: The table contains network prefixes and their corresponding AS number and country code:
+-----------------+-------+--------+
| prefix | asn | cca2 |
+=================+=======+========+
| 202.79.32.0/20 | 17501 | NP |
+-----------------+-------+--------+
| 2620:0:870::/48 | 3856 | US |
+-----------------+-------+--------+
| 2a02:6b8:1::/48 | 13238 | RU |
+-----------------+-------+--------+
| 2001:db8::/32 | 65536 | ZZ |
+-----------------+-------+--------+
When using this type of layout, the structure must have a composite key.
Example:
<structure>
<key>
<attribute>
<name>prefix</name>
<type>String</type>
</attribute>
</key>
<attribute>
<name>asn</name>
<type>UInt32</type>
<null_value />
</attribute>
<attribute>
<name>cca2</name>
<type>String</type>
<null_value>??</null_value>
</attribute>
...
The key must have only one String type attribute that contains an allowed IP prefix. Other types are not supported yet.
For queries, you must use the same functions (dictGetT
with a tuple) as for dictionaries with composite keys:
dictGetT('dict_name', 'attr_name', tuple(ip))
The function takes either UInt32
for IPv4, or FixedString(16)
for IPv6:
dictGetString('prefix', 'asn', tuple(IPv6StringToNum('2001:db8::1')))
Other types are not supported yet. The function returns the attribute for the prefix that corresponds to this IP address. If there are overlapping prefixes, the most specific one is returned.
Data is stored in a trie
. It must completely fit into RAM.