mirror of
https://github.com/ClickHouse/ClickHouse.git
synced 2024-10-12 03:20:51 +00:00
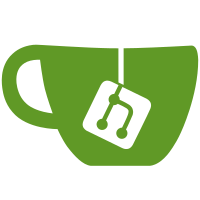
* Some improvements for introduction/performance.md
* Minor improvements for example_datasets
* Add website/package-lock.json to .gitignore
* YT paragraph was badly outdated and there is no real reason to write a new one
* Use weird introduction article as a starting point for F.A.Q.
* Some refactoring of first half of ya_metrika_task.md
* minor
* Weird docs footer bugfix
* Forgotten redirect
* h/v scrollbars same size in docs
* CLICKHOUSE-3831: introduce security changelog
* A bit more narrow tables on docs front page
* fix flag in ru docs
* Save some space in top level of docs ToC
* Capitalize most words in titles of docs/en/
* more docs scrollbar fixes
* fix incorrect merge
* fix link
* fix switching languages in single page docs mode
* Update mkdocs & mkdocs-material + unminify javascript
* cherrypick 17e18d1ecc
13 lines
1.6 KiB
Markdown
13 lines
1.6 KiB
Markdown
# General Questions
|
|
|
|
## Why Not Use Something Like MapReduce?
|
|
|
|
We can refer to systems like MapReduce as distributed computing systems in which the reduce operation is based on a distributed sort. The most common opensource solution of this kind is [Apache Hadoop](http://hadoop.apache.org), while Yandex internally uses it's own MapReduce implementation — YT.
|
|
|
|
The systems of this kind are not suitable for online queries due to their high latency. In other words, they can't be used as the back-end for a web interface.
|
|
|
|
Distributed sorting isn't the best way to perform reduce operations if the result of the operation and all the intermediate results (if there are any) are located in the RAM of a single server, which is usually the case for online queries. In such a case, a hash table is the optimal way to perform reduce operations. A common approach to optimizing MapReduce tasks is pre-aggregation (partial reduce) using a hash table in RAM. The user performs this optimization manually.
|
|
Distributed sorting is one of the main causes of reduced performance when running simple MapReduce tasks.
|
|
|
|
Most MapReduce implementations allow executing any code on the cluster. But a declarative query language is better suited to OLAP in order to run experiments quickly. For example, Hadoop has Hive and Pig. Also consider Cloudera Impala, Shark (outdated) for Spark, and Spark SQL, Presto, and Apache Drill. Performance when running such tasks is highly sub-optimal compared to specialized systems, but relatively high latency makes it unrealistic to use these systems as the backend for a web interface.
|