mirror of
https://github.com/ClickHouse/ClickHouse.git
synced 2024-11-17 05:03:20 +00:00
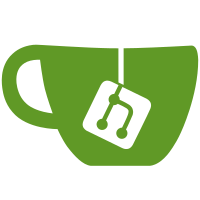
* CLICKHOUSE-4063: less manual html @ index.md * CLICKHOUSE-4063: recommend markdown="1" in README.md * CLICKHOUSE-4003: manually purge custom.css for now * CLICKHOUSE-4064: expand <details> before any print (including to pdf) * CLICKHOUSE-3927: rearrange interfaces/formats.md a bit * CLICKHOUSE-3306: add few http headers * Remove copy-paste introduced in #3392 * Hopefully better chinese fonts #3392 * get rid of tabs @ custom.css * Apply comments and patch from #3384 * Add jdbc.md to ToC and some translation, though it still looks badly incomplete * minor punctuation * Add some backlinks to official website from mirrors that just blindly take markdown sources * Do not make fonts extra light * find . -name '*.md' -type f | xargs -I{} perl -pi -e 's//g' {} * find . -name '*.md' -type f | xargs -I{} perl -pi -e 's/ sql/g' {} * Remove outdated stuff from roadmap.md * Not so light font on front page too * Refactor Chinese formats.md to match recent changes in other languages
1.4 KiB
1.4 KiB
常见问题
为什么不使用MapReduce之类的产品呢?
我们可以将MapReduce这类的系统称为分布式计算系统,其reduce操作基于分布式排序。其中最常见的开源解决方案是Apache Hadoop。 Yandex使用他们的内部解决方案YT。
这些系统不适合在线查询,因为它们的延迟高。换句话说,它们不能用作Web接口的后端服务。这些系统对于实时数据更新是没有用的。如果操作的结果和所有中间结果(如果有的话)位于单个服务器的内存中,则分布式排序不是执行reduce操作的最佳方式,但这通常是在线查询的情况。在这种情况下,哈希表是执行reduce操作的最佳方式。优化map-reduce任务的常用方法是使用内存中的哈希表进行预聚合(部分reduce),用户手动执行此优化操作。分布式排序是运行简单map-reduce任务时性能降低的主要原因之一。
大多数MapReduce系统允许您在集群上执行任意代码。但是,声明性查询语言更适合OLAP,以便快速运行实验。例如,Hadoop包含Hive和Pig,Cloudera Impala或Shark(过时)for Spark,以及Spark SQL、Presto和Apache Drill。与专业系统相比,运行此类任务时的性能非常不理想,所以将这些系统用作Web接口的后端服务是不现实的,因为延迟相对较高。